FCNN
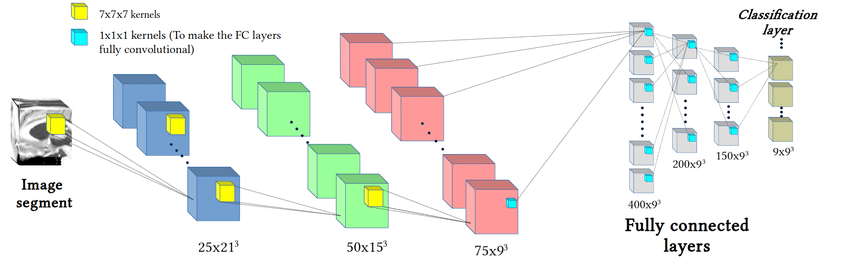
In the realm of artificial intelligence and computer vision, the emergence of Fully Convolutional Neural Networks (FCNN) has marked a paradigm shift, unlocking new frontiers in image processing, semantic segmentation, and beyond. FCNNs, with their ability to process variable-sized inputs and produce pixel-wise predictions, have revolutionized the way machines perceive and interpret visual data. In this article, we delve into the intricacies of FCNNs, exploring their architecture, applications, and the transformative impact they’ve had across various domains.
Understanding FCNNs:
At its core, a Fully Convolutional Neural Network is an extension of traditional convolutional neural networks (CNNs) designed for pixel-wise prediction tasks. Unlike traditional CNNs that are primarily used for classification tasks, FCNNs preserve spatial information throughout the network, enabling them to generate dense predictions at the pixel level.
The architecture of an FCNN typically comprises several convolutional layers followed by upsampling layers. These upsampling layers are crucial for increasing the spatial resolution of the feature maps, allowing the network to generate predictions at the original input resolution. Additionally, FCNNs often incorporate skip connections to fuse low-level and high-level features, facilitating better segmentation performance.
Applications of FCNNs:
- Semantic Segmentation: One of the primary applications of FCNNs is semantic segmentation, where the goal is to partition an image into semantically meaningful regions. FCNNs excel in this task by producing pixel-wise predictions, enabling precise delineation of objects and their boundaries. Applications include medical image analysis, autonomous driving, and scene understanding in robotics.
- Instance Segmentation: While semantic segmentation assigns a class label to each pixel, instance segmentation takes a step further by distinguishing individual object instances within the same class. FCNNs, with their ability to capture fine-grained details, have demonstrated remarkable performance in instance segmentation tasks, facilitating applications like object counting, tracking, and image-based measurements.
- Image-to-Image Translation: FCNNs have been leveraged for various image-to-image translation tasks, such as style transfer, image super-resolution, and image synthesis. By learning the mapping between input and output domains, FCNNs can transform images while preserving important visual characteristics. This has wide-ranging applications in creative industries, graphic design, and digital content generation.
- Medical Image Analysis: In the field of healthcare, FCNNs have emerged as indispensable tools for medical image analysis tasks, including tumor detection, organ segmentation, and disease diagnosis. Their ability to process complex visual data and extract meaningful features has paved the way for more accurate and efficient medical diagnostics, ultimately improving patient outcomes.
- Remote Sensing and Geospatial Analysis: FCNNs play a crucial role in remote sensing applications, such as land cover classification, urban mapping, and environmental monitoring. By analyzing satellite imagery and aerial photographs, FCNNs can extract valuable insights about land use, vegetation coverage, and natural disasters, aiding in urban planning, agriculture, and disaster management efforts.
Challenges and Future Directions:
Despite their remarkable achievements, FCNNs are not without challenges. One of the primary concerns is the computational complexity associated with processing high-resolution images, which can hinder real-time deployment in resource-constrained environments. Addressing this challenge requires ongoing research into model optimization techniques, efficient architectures, and hardware acceleration strategies.
Furthermore, ensuring the robustness and generalization of FCNNs across diverse datasets and real-world scenarios remains a pressing concern. The development of more robust training procedures, data augmentation techniques, and domain adaptation methods is crucial for enhancing the performance and reliability of FCNNs in practical applications.
Looking ahead, the future of FCNNs holds promise for continued advancements in image processing, computer vision, and beyond. As researchers explore novel architectures, learning algorithms, and interdisciplinary applications, FCNNs are poised to further expand their impact across various domains, shaping the future of artificial intelligence and transforming the way we perceive and interact with visual data.
Conclusion:
Fully Convolutional Neural Networks have emerged as powerful tools for pixel-wise prediction tasks, revolutionizing image processing, semantic segmentation, and a myriad of other applications. With their ability to preserve spatial information, generate dense predictions, and capture fine-grained details, FCNNs have ushered in a new era of machine perception and understanding. As research in this field continues to advance, FCNNs are poised to drive innovation, tackle complex challenges, and unlock new possibilities across diverse domains, reaffirming their status as indispensable components of the modern AI toolkit.